Metrics, evidence and audit in text analytics
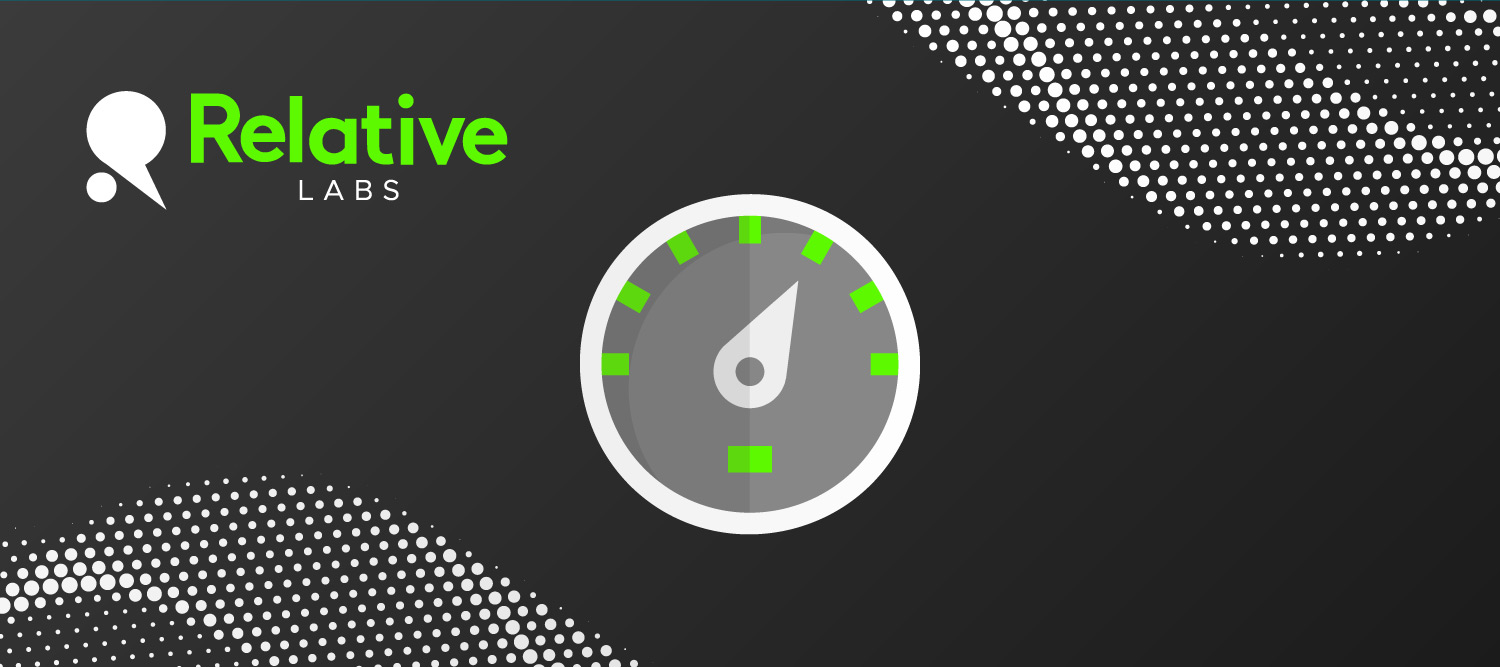
AI is causing the corporate world to reimagine what can be done with processes, creativity, and business models. Business analytics is no exception, as practitioners, vendors and consultants all try and predict what skills and technologies they will need to stay relevant in the future.
At Relative Insight, we’ve seen the Gartner Hype Cycle play out en masse in a record time across the industry. Different companies are at different parts of their journey in understanding exactly how Large Language Models (LLMs) will affect their roles, and what are their benefits and disadvantages.
What are the goals of business analytics?
We are engaging in conversations with our customers based on first principles, and then we are shaping the technology to fit around the goals we want to achieve. This is important to bear in mind when assessing technology.
What are analytics for? What is the goal? Why do businesses spend money on business analytics?
- To guide effective business decisions
- To give confidence to the business decisions already taken
- To predict the future
- To track crucial trends for the business
- To inspire new ways of thinking
- To align companies around what matters
It won’t come as a surprise to hear that Relative Insight brings value from unstructured text into business analytics. We truly believe in the power of text to bring a significant shift in the way companies understand their customers, their services, their employees and society.
But, what we have learned is that to become a trusted source of business intelligence, three capabilities are necessary to make an impact on a business – and all of these capabilities are lacking in LLMs when considered individually.
These are Metrics, Evidence and Audit.
Understanding metrics
Unstructured text holds a lot of promise in analytics and has now been catapulted up the corporate agenda by the publicity around LLMs. But this begs the question of why unstructured text was not higher up the agenda in the first place. If it can bring great value to businesses then why is it not considered a standard part of business reporting?
This can be partially explained by the vague outputs that text analytics software has historically produced. The output of any analytical exercise has to engage its audience and stakeholders quickly to allow measurement and business alignment.
You can’t create business alignment out of a word cloud. To create alignment, understanding and action you need to have metrics.
Metrics empower customers to perform measurement, alignment, tracking and communication. This is why, for example, NPS has been adopted so widely; a sophisticated analysis expressed as a metric. Without measurable metrics, it is much harder to have your analysis communicated, understood and acted upon.
Large Language Models and text analytics
While LLMs are creating a lot of headline interest in text analytics, they’re only being used to generate text summarizations. Although this initially seemed captivating to stakeholders, we are seeing the interest wain. Having a paragraph of unstructured text as the output of your AI is seductive in the short term. But it quickly falls into the trap of not providing any alignment, tracking or easy communication.
Relative Insight’s approach focuses on the generation of sophisticated metrics from unstructured text data. This is why companies such as Comcast, HSBC, and MetLife use our software, turning unstructured text from consumer analytics, customer experience, and employee experience into metrics for business alignment.
Of course, we also use LLMs and generative AI in our solutions. But as a professional analyst, which one would you rather have: a summarization of text or a summarization of text with critical metrics embedded in the output?
The importance of evidence
It has been well-documented that LLMs are black boxes. You cannot pick apart the rationale that they have used to come to an analysis or an output. Similarly, they are known to have hallucinations, where a conclusion is false, or the evidence they provide is made up.
Generally, this is not always a bad thing and it largely depends on your use case. If you are using generative AI to enhance your ad copy, and it proves effective in boosting revenue, then do you really care about the rationale used? Perhaps not.
However, if the decisions you are making based on the analytic output are non-reversible or have high consequences, then you may want to have evidence of what recommendations have been given.
Let’s walk through an example of a major training and process transformation project linked to customer experience. You have performance metrics for customer experience teams and you also have unstructured text in the form of customer surveys, chat transcripts and call transcripts. The text data provides critical intelligence on how to answer queries better, what effect training has on customer satisfaction, etc.
Before committing to an expensive and hard-to-reverse project, the rationale for any analytic conclusions needs to be examined. It would be unwise to simply “trust the LLM” in such a critical function.
Furthermore, the evidence and justification need to be understood. This is where a proper chain of evidence for recommendations is practical, with the evidence stemming from the metrics the AI is basing its decisions on.
Transform your text data into quantifiable metrics with the added benefits of an evidence trail and audit capabilities
A new approach to audit
In some cases, there may also be a requirement to audit why certain decisions have been taken. This includes, for example, regulatory purposes.
Some illustrations of the power of LLMs refer to the use case of pulling together various R&D work streams and documents to provide a summarization for different stakeholders.
Although this is a good application for LLMs, it will be crucial that some kind of audit capability is available in the decision-making process. Given the known issues around the black box capability of LLMs and the hallucination effects, certain use cases might necessitate a mandatory audit trail.
For example, consider the consolidation of pharmaceutical trial results into summarization for healthcare practitioners or the formulation of retirement guidance recommendations. We have already seen legal submissions being subject to audit. As the regulatory framework gradually starts to catch up with AI advances, this will become a mandatory capability in some application areas.
Audit capability needs to come from the metrics in the original data. Producing a black box summary here will fall foul of regulatory concerns. Therefore, businesses should proactively prepare for this requirement by designing their analytic systems to incorporate capabilities for auditing.
As AI becomes more prevalent, the scrutiny on it will also increase. Metrics, Evidence and Audit will become table stakes for analytics practitioners. Adopting these now will see leaders in the space quickly capitalize on the corporate interest in the topic.
Leave a Comment