5 key features of text analysis tools
Are you still relying on painstaking manual approaches for analyzing text from open-ended survey responses, online reviews, customer service transcripts, and social media conversations?
Or worse, are you neglecting these valuable sources of insight altogether?
When done well, text analysis – also referred to as text mining – is the ultimate way to analyze free text data and find the insights hiding within it.
Text analysis tools are the rockstar of modern qualitative research, offering teams the ability to uncover objective and actionable insights while becoming more efficient with analysts’ time by reducing the need for manual analysis.
These questions are crucial because not all text analysis software is born equal.
In this guide, we’ll dive into the five key features anyone looking to add a text analytics platform to their BI toolkit should be familiar with.
What are the key features of text analysis tools?
The feature sets of text data mining software can differ widely, but most include a combination of word frequency analysis, topical text classification, emotion or sentiment analysis, visualizations and data management.

Let’s cover off each in more detail…
Word frequency analysis
The most basic form of text analysis, word frequency analysis does exactly what it says on the label: it analyzes text data to identify the most commonly used words (or in some cases, phrases).
It’s a way of understanding the dominant words in a data set.
The results are often displayed in the form of a frequency table or word cloud like the one below, a visual format that has become synonymous with social media listening tools over the past decade.
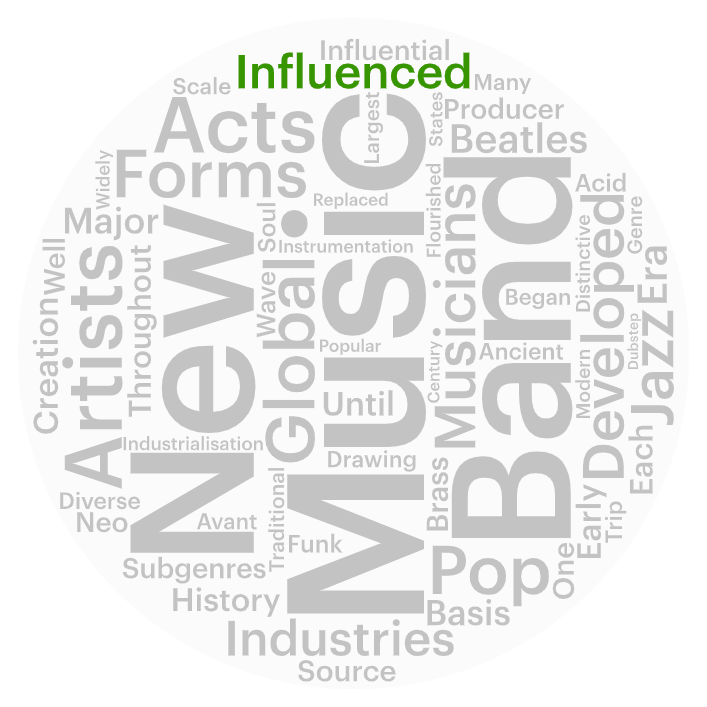
The problem with word clouds i.e. word frequency analysis is that they tend to surface topic-specific vocabulary that is obvious or expected.
In the example above, we see the words ‘band’, music’ and ‘artists’ featured prominently. But it’s not particularly insightful knowing that people discussing concerts on social media are using these words – in fact, it would be hard to talk about the topic without them!
Because of this, word clouds struggle to see past the headlines of what is happening in your data and get into the nuance of what audiences are saying.
Topical text classification
Where word frequency can offer basic analysis but sometimes bog you down in more detail than is helpful, topical text classification offers a way to efficiently unearth the key themes within your text data.
Linguistic analysis is (frustratingly) never as simple as saying Word X belongs to Topic Y, as words can take on different meanings in different contexts.
Let’s imagine you were conducting a social media analysis to understand your audience’s latest thoughts on your new SaaS product.
One post might say “an easier service you’d struggle to find” whereas another might say “I thought this software was supposed to make my life easier?”
Basic text classification tools would struggle to distinguish between these two references of “easier”. But AI-powered topical text classification tools can make a more nuanced and reliable judgement on how a particular word is being used.
The best text analysis tools typically offer some combination of out-of-the-box topical classification like this, as well as the ability to define and analyze custom, user-defined topics.
Stop wasting time with word clouds!
Sentiment analysis of text vs. emotional analysis
Understanding how people feel about your brand, products, competitors, and related topics is important.
Many free text analysis tools use sentiment analysis to do this, but the best text analysis tool takes it a step further with emotional text analysis.
While a popular approach, sentiment analysis just isn’t nuanced enough to really help you understand target audiences’ emotions.
Sentiment tools attempt to classify text as positive, negative, or neutral. This can be helpful on a basic level, but it’s not sophisticated enough to tap into the real emotions at play.
Text analysis tools like Relative Insight, however, can help you gain a deeper emotional understanding of your audiences.
While sentiment tools might tell you your text is mostly positive, emotional text analysis can, for example, help you distinguish between joy, contentment, or hope.
Take an example hotel review where a guest has said “I wish I could say that the hotel host was friendly but in reality, she was a bit too keen if you catch my drift.”
A sentiment analysis tool would likely pick out words like “friendly” and “keen” and label this review as positive.
An emotional free text analysis tool like Relative Insight would be better equipped to detect the underlying frustration by distinguishing between “keen” and “a bit too keen”.
Text analysis visualizations
Beyond helping you understand what and how people are talking about topics relevant to your business, text analysis tools also offer different types of text analysis visualization that allow you to understand what’s happening in your data at a glance.
Visualizations are useful for spotting trends in your data that otherwise would have gone unnoticed and can enable you to take corrective action before emerging issues become full-blown problems.
In Relative Insight, Heartbeat charts are a visualization tool that allow you to track changes in the prevalence of key topics in customer feedback and social media conversations over time.
A retailer might use such a tool to track changes in customer survey responses around product quality, affordability, customer service and delivery issues.
Heatmaps, another of our qualitative data visualization examples, helps you quickly identify which data sets have the most and least in common.
For example, the Heatmap below shows us the UK pizza chains that are most and least differentiated in their brand messaging.

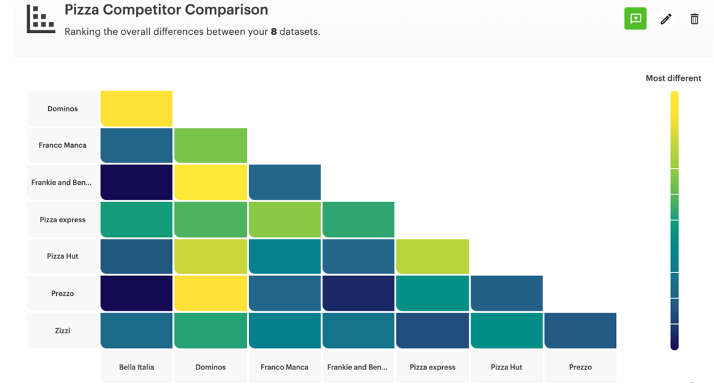
Many text analysis tools can feed visualizations directly into BI tools and dashboards like Tableau or Power BI, making it seamless and pain-free to integrate text analytics with your existing processes and reports.
This makes it easy to communicate your findings in intriguingly simple ways that everyone can understand.
Visualize trends in customer feedback in seconds
Data management
Text mining software tends to offer a range of options when it comes to uploading data for analysis.
This can involve a simple upload from .csv or Excel files or joining up data sources directly using a text analytics API connection. Such connections automatically push your data into your text analytics platform for analysis, without any need for manual intervention.
Most text mining tools will then provide a suite of tools to help you filter, clean and segment your data.
Hassle-free data management means your teams can spend more time on analysis and less time stressing over data.
Advice for finding the right text analysis tool
It’s important to remember that word frequency and sentiment analysis can be valuable tools, but are just two pieces of the puzzle when it comes to getting insights from your text data.
The presence of topical text classification, emotional analysis and visualizations (other than word clouds) are good indicators of a more sophisticated offering that will help you move beyond the headlines in your data.
Last, but not least, it’s crucial to consider the ease with which you can get data from collection tools into your text analysis tool. The ability to take the stress out of data management can be the difference between good text analysis tools and a great one.
Ultimately, to do text analysis well, you need a platform that combines the best of these features, allowing you to surface valuable insights from your qualitative data at scale.