Driving business value from unstructured text
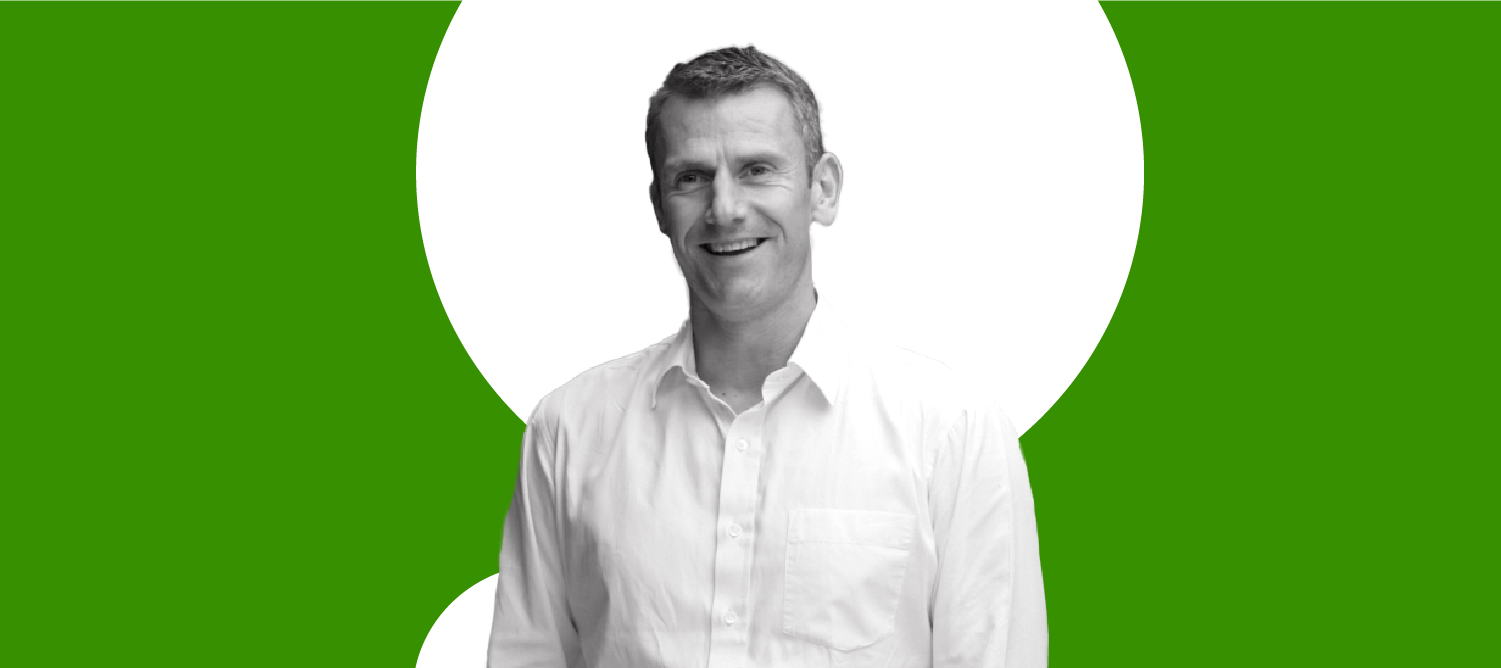
At Relative Insight, we’ve always held the conviction that language provides a critical source of insight that’s often overlooked, and our mission is to help our customers drive value from all those language data sources that they’ve got floating around in their organisations, not doing much.
Typical examples of what’s usually found in this ‘data soup’ are the results of open-ended surveys, customer reviews, conversations about you or your brand on social media and forums, and of course customer interactions that can only go so far.
It’s one thing to analyse an element of all of this data information, and get one-time value out of it, but it’s quite another to squeeze all the juice out of it, and have it work really hard for you. The “one and done” approach to data analysis is often a typical model used by insights organisations but actually – and let me continue with my juice metaphor – you’ll find that by analysing something once, you’ll find you’re missing out on all that delicious fruit, and its life-affirming qualities.
So for example, you conduct a major open-ended survey exercise, you analyse those responses to an extent and it gets put into a report, then that’s it. That data still exists and could be sliced and diced (fruit again) in different ways, but it rarely is, it’s just left to rot.
We want to transform this thinking. Our customers are able to view language as a strategic asset which is stored and analysed in different ways, depending on the business problem being examined. This centralised storage and analysis methodology allows them to get lots of different sorts of value from that one set of language data that they may have only used once before.
Relative Insight recently released some new server architecture which makes this even easier to do, and we’ve already seen that our customers are seeing at least a five times increase in the value they get from their data. What I mean by this, is that when any one set of language data is uploaded, it isn’t just analysed once, rather it’s used in at least five different ways to provide five different sets of analysis and insights.
For example, when the survey results asking contributors to give their opinions on a specific brand are uploaded into our platform, they may be analysed in multiple ways – and we can answer lots of different questions, including:
- How are different customer groups or demographics responding to the questions?
- How do these responses compare to other survey results in the past – what’s changed over time?
- What happens when responses are split by NPS scores – so what’s the differences between “neutral” and “would recommend” customer comments?
- How do these survey comments compare to other forms of consumer feedback, including those collected from social media, forum conversations and review sites?
- If we split the responses by brand mentions for example, how do comments about our brand compare to our top three competitors?
So, by using language comparison as a strategic way to analyse your data, you’ll find that your orange has a long shelf-life, rather than going off after a couple of days.
If you would like to see our technology in action, get in touch and together we’ll put it through its paces.